Weaknesses of Genetic Algorithms with Precedence Preservative Crossover and Mutation in Disassembly Sequence Optimization
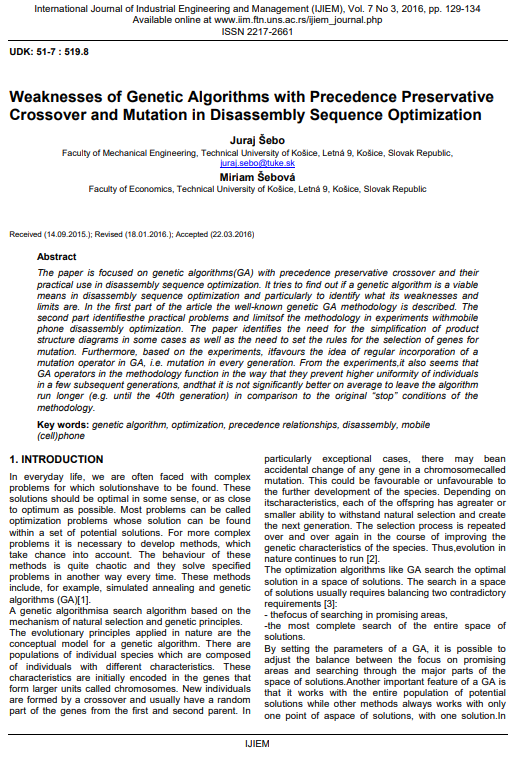
Published 2016-09-30
abstract views: 20 // FULL TEXT ARTICLE (PDF): 0
Keywords
- genetic algorithm,
- optimization,
- precedence relationships,
- disassembly,
- mobile (cell)phone
How to Cite
Copyright (c) 2023 International Journal of Industrial Engineering and Management

This work is licensed under a Creative Commons Attribution 4.0 International License.
Abstract
The paper is focused on genetic algorithms(GA) with precedence preservative crossover and their practical use in disassembly sequence optimization. It tries to find out if a genetic algorithm is a viable means in disassembly sequence optimization and particularly to identify what its weaknesses and limits are. In the first part of the article the well-known genetic GA methodology is described. The second part identifiesthe practical problems and limitsof the methodology in experiments withmobile phone disassembly optimization. The paper identifies the need for the simplification of product structure diagrams in some cases as well as the need to set the rules for the selection of genes for mutation. Furthermore, based on the experiments, itfavours the idea of regular incorporation of a mutation operator in GA, i.e. mutation in every generation. From the experiments,it also seems that GA operators in the methodology function in the way that they prevent higher uniformity of individualsin a few subsequent generations, andthat it is not significantly better on average to leave the algorithm run longer (e.g. until the 40th generation) in comparison to the original “stop” conditions of the methodology.
Article history: Received (14.09.2015); Revised (18.01.2016); Accepted (22.03.2016)