Optimizing Smart Manufacturing Processes and Human Resource Management through Machine Learning Algorithms
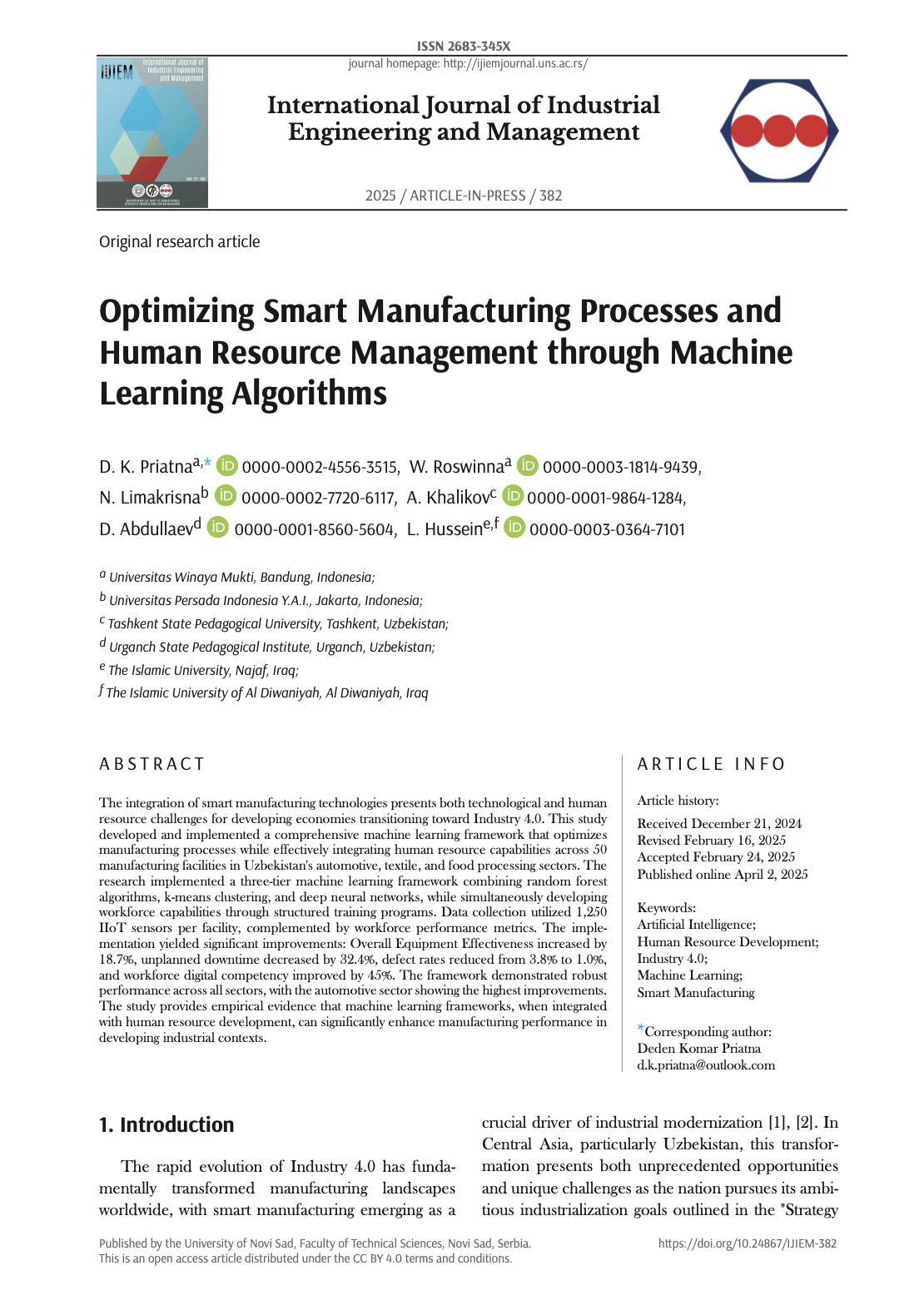
Published 2025-06-01
abstract views: 355 // FULL TEXT ARTICLE (PDF): 59
Keywords
- Artificial Intelligence,
- Human Resource Development,
- Industry 4.0,
- Machine Learning,
- Smart Manufacturing
How to Cite
Copyright (c) 2025 International Journal of Industrial Engineering and Management

This work is licensed under a Creative Commons Attribution 4.0 International License.
Abstract
The integration of smart manufacturing technologies presents both technological and human resource challenges for developing economies transitioning toward Industry 4.0. This study developed and implemented a comprehensive machine learning framework that optimizes manufacturing processes while effectively integrating human resource capabilities across 50 manufacturing facilities in Uzbekistan's automotive, textile, and food processing sectors. The research implemented a three-tier machine learning framework combining random forest algorithms, k-means clustering, and deep neural networks, while simultaneously developing workforce capabilities through structured training programs. Data collection utilized 1,250 IIoT sensors per facility, complemented by workforce performance metrics. The implementation yielded significant improvements: Overall Equipment Effectiveness increased by 18.7%, unplanned downtime decreased by 32.4%, defect rates reduced from 3.8% to 1.0%, and workforce digital competency improved by 45%. The framework demonstrated robust performance across all sectors, with the automotive sector showing the highest improvements. The study provides empirical evidence that machine learning frameworks, when integrated with human resource development, can significantly enhance manufacturing performance in developing industrial contexts.
Article history: Received (December 21, 2024); Revised (February 16, 2025); Accepted (February 24, 2025); Published online (April 2, 2025)