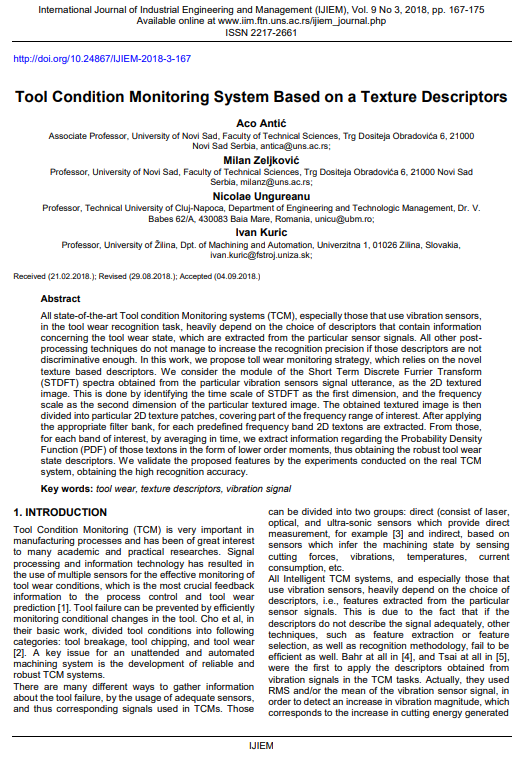
Published 2018-09-30
abstract views: 6 // FULL TEXT ARTICLE (PDF): 0
Keywords
- tool wear,
- texture descriptors,
- vibration signal
How to Cite
Copyright (c) 2023 International Journal of Industrial Engineering and Management

This work is licensed under a Creative Commons Attribution 4.0 International License.
Abstract
All state-of-the-art Tool condition Monitoring systems(TCM), especially those that use vibration sensors, in the tool wear recognition task, heavily depend on the choice of descriptors that contain information concerning the tool wear state, which are extracted from the particular sensor signals. All other post-processing techniques do not manage to increase the recognition precision if those descriptors are not discriminative enough. In this work, we propose toll wear monitoring strategy, which relies on the novel texture based descriptors. We consider the module of the Short Term Discrete Furrier Transform (STDFT) spectra obtained from the particular vibration sensors signal utterance, as the 2D textured image. This is done by identifying the time scale of STDFT as the first dimension, and the frequency scale as the second dimension of the particular textured image. The obtained textured image is then divided into particular 2D texture patches, covering part of the frequency range of interest. After applying the appropriate filter bank, for each predefined frequency band 2D textons are extracted. From those, for each band of interest, by averaging in time, we extract information regarding the Probability Density Function (PDF)of those textons in the form of lower order moments, thus obtaining the robust tool wear state descriptors. We validate the proposed features by the experiments conducted on the real TCM system, obtaining the high recognition accuracy.
Article history: Received (21.02.2018); Revised (29.08.2018); Accepted (04.09.2018)