A framework for unsupervised learning and predictive maintenance in Industry 4.0
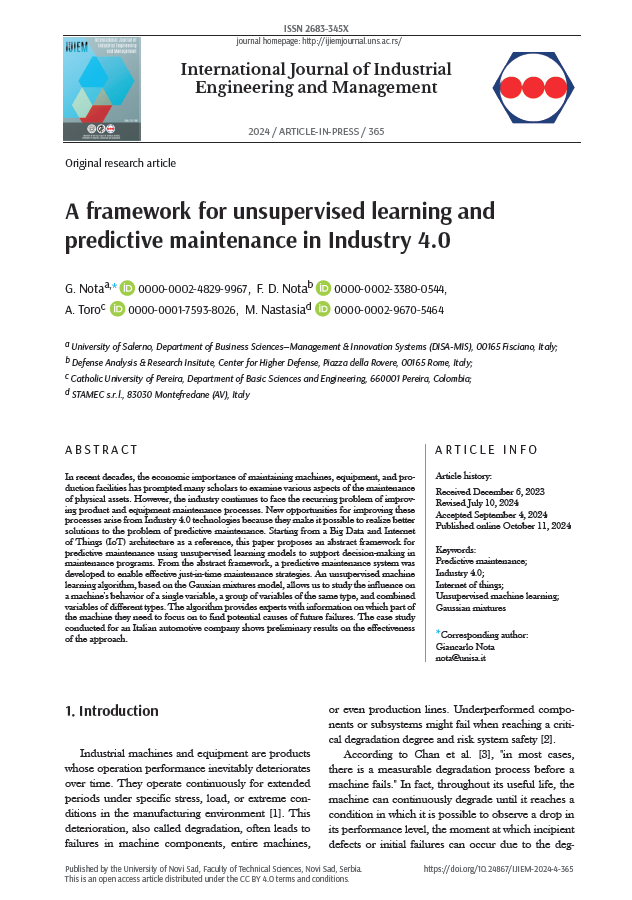
Published 2024-10-11
abstract views: 87 // FULL TEXT ARTICLE (PDF): 50
Keywords
- Predictive maintenance,
- Industry 4.0,
- Internet of things,
- Unsupervised machine learning,
- Gaussian mixtures
How to Cite
Copyright (c) 2024 International Journal of Industrial Engineering and Management

This work is licensed under a Creative Commons Attribution 4.0 International License.
Abstract
In recent decades, the economic importance of maintaining machines, equipment, and production facilities has prompted many scholars to examine various aspects of the maintenance of physical assets. However, the industry continues to face the recurring problem of improving product and equipment maintenance processes. New opportunities for improving these processes arise from Industry 4.0 technologies because they make it possible to realize better solutions to the problem of predictive maintenance. Starting from a Big Data and Internet of Things (IoT) architecture as a reference, this paper proposes an abstract framework for predictive maintenance using unsupervised learning models to support decision-making in maintenance programs. From the abstract framework, a predictive maintenance system was developed to enable effective just-in-time maintenance strategies. An unsupervised machine learning algorithm, based on the Gauxian mixtures model, allows us to study the influence on a machine's behavior of a single variable, a group of variables of the same type, and combined variables of different types. The algorithm provides experts with information on which part of the machine they need to focus on to find potential causes of future failures.
Article history: Received (December 6, 2023); Revised (July 10, 2024); Accepted (September 4, 2024); Published online (October 11, 2024)